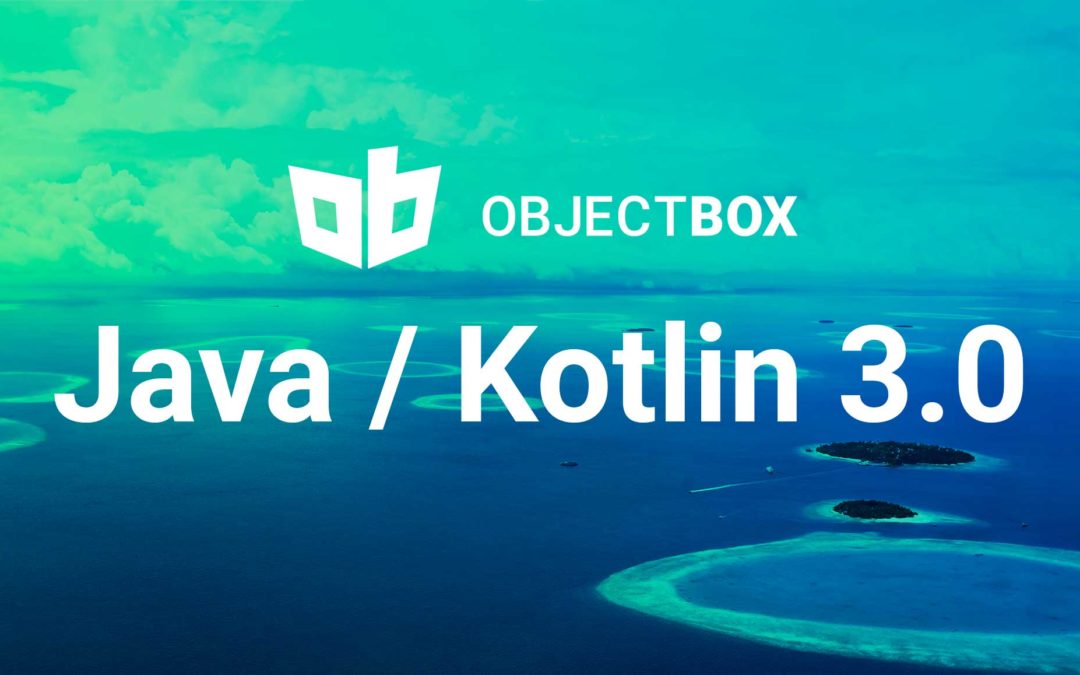
ObjectBox Database Java / Kotlin 3.0 + CRUD Benchmarks
We love our Java / Kotlin community ❤️ who have been with us since day one. So, with today’s post, we’re excited to share a feature-packed new major release for Java Database alongside CRUD performance benchmarks for MongoDB Realm, Room (SQLite) and ObjectBox.
What is ObjectBox?
ObjectBox is a high performance database and an alternative to SQLite and Room. ObjectBox empowers developers to persist objects locally on Mobile and IoT devices. It’s a NoSQL ACID-compliant object database with an out-of-the-box Data Sync providing fast and easy access to decentralized edge data (Early Access).
New Query API
A new Query API is available that works similar to our existing Dart/Flutter Query API and makes it easier to create nested conditions:
1 2 3 4 5 6 7 8 9 10 11 12 13 14 15 16 17 |
// Kotlin // equal AND (less OR oneOf) val query = box.query( User_.firstName equal "Joe" and (User_.age less 12 or (User_.stamp oneOf longArrayOf(1012)))) .order(User_.age) .build() // Java // equal AND (less OR oneOf) Query<User> query = box.query( User_.firstName.equal("Joe") .and(User_.age.less(12) .or(User_.stamp.oneOf(new long[]{1012})))) .order(User_.age) .build(); |
In Kotlin, the condition methods are also available as infix functions. This can help make queries easier to read:
1 |
val query = box.query(User_.firstName equal "Joe").build() |
Unique on conflict replace strategy
One unique property in an @Entity can now be configured to replace the object in case of a conflict (“onConflict”) when putting a new object.
1 2 3 4 5 6 7 8 9 10 11 12 13 14 15 16 17 18 19 |
// Kotlin @Entity data class Example( @Id var id: Long = 0, @Unique(onConflict = ConflictStrategy.REPLACE) var uniqueKey: String? = null ) // Java @Entity public class Example { @Id public long id; @Unique(onConflict = ConflictStrategy.REPLACE) String uniqueKey; } |
This can be helpful when updating existing data with a unique ID different from the ObjectBox ID. E.g. assume an app that downloads a list of playlists where each has a modifiable title (e.g. “My Jam”) and a unique String ID (“playlist-1”). When downloading an updated version of the playlists, e.g. if the title of “playlist-1” has changed to “Old Jam”, it is now possible to just do a single put with the new data. The existing object for “playlist-1” is then deleted and replaced by the new version.
Built-in string array and map support
String array or string map properties are now supported as property types out-of-the-box. For string array properties it is now also possible to find objects where the array contains a specific item using the new containsElement
condition.
1 2 3 4 5 6 7 8 9 10 11 12 13 14 15 16 17 18 19 20 21 22 23 24 |
// Kotlin @Entity data class Example( @Id var id: Long = 0, var stringArray: Array<String>? = null, var stringMap: MutableMap<String, String>? = null ) // matches [“first”, “second”, “third”] box.query(Example_.stringArray.containsElement(“second”)).build() // Java @Entity public class Example { @Id public long id; public String[] stringArray; public Map<String, String> stringMap; } // matches [“first”, “second”, “third”] box.query(Example_.stringArray.containsElement(“second”)).build(); |
Kotlin Flow, Android 12 and more
Kotlin extension functions were added to obtain a Flow from a BoxStore or Query:
1 |
val flow = box.query().subscribe().toFlow() |
Data Browser has added support for apps targeting Android 12.
For details on all changes, please check the ObjectBox for Java changelog.
Room (SQLite), Realm & ObjectBox CRUD performance benchmarks
We compared against the Android databases, MongoDB Realm and Room (on top of SQLite) and are happy to share that ObjectBox is still faster across all four major database operations: Create, Read, Update, Delete.
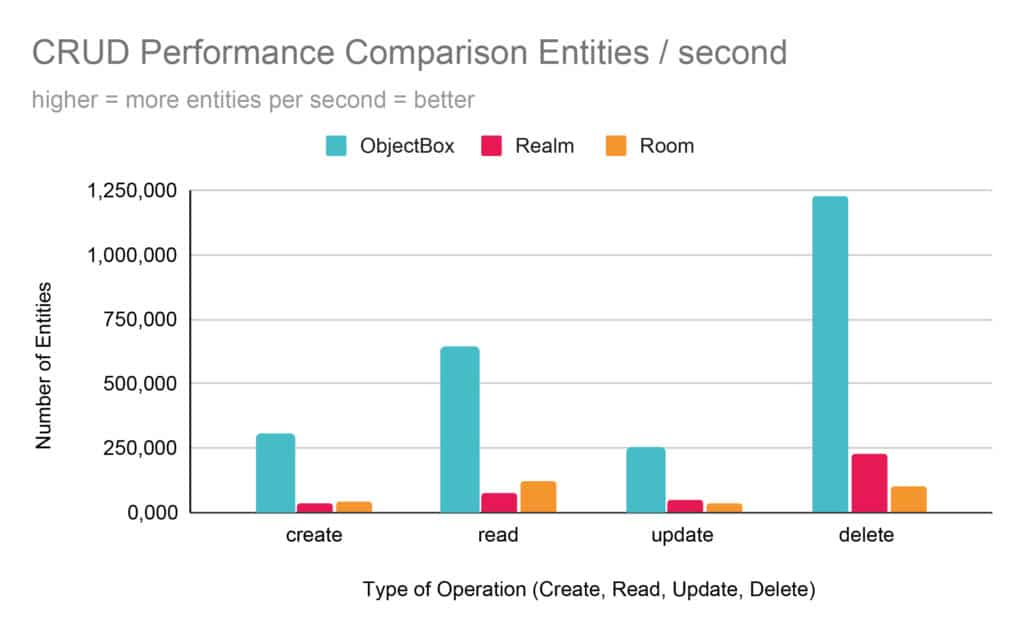
We benchmarked ObjectBox along with Room 2.3.0 using SQLite 3.22.0 and MongoDB Realm 10.6.1 on an Samsung Galaxy S9+ (Exynos) mobile phone with Android 10. All benchmarks were run 10+ times and no outliers were discovered, so we used the average for the results graph above. Find our open source benchmarking code on GitHub and as always: feel free to check them out yourself. More to come soon, follow us on Twitter or sign up to our newsletter to stay tuned (no spam ever!).
Using a fast on-device database matters
A fast local database is more than just a “nice-to-have.” It saves device resources, so you have more resources (CPU, Memory, battery) left for other resource-heavy operations. Also, a faster database allows you to keep more data locally with the device and user, thus improving privacy and data ownership by design. Keeping data locally and reducing data transferal volumes also has a significant impact on sustainability.
Sustainable Data Sync
Some data, however, you might want or need to synchronize to a backend. Reducing overhead and synchronizing data selectively, differentially, and efficiently reduces bandwidth strain, resource consumption, and cloud / Mobile Network usage – lowering the CO2 emissions too. Check out ObjectBox Data Sync, if you are interested in an out-of-the-box solution.
Get Started with ObjectBox for Java / Kotlin Today
ObjectBox is free to use and you can get started right now via this getting-started article, or follow this video.
Already an ObjectBox Android database user and ready to take your application to the next level? Check out ObjectBox Data Sync, which solves data synchronization for edge devices, out-of-the-box. It’s incredibly efficient and (you guessed it) superfast 😎
We ❤️ your Feedback
We believe, ObjectBox is super easy to use. We are on a mission to make developers’ lives better, by building developer tools that are intuitive and fun to code with. Now it’s your turn: let us know what you love, what you don’t, what do you want to see next? Share your feedback with us, or check out GitHub and up-vote the features you’d like to see next in ObjectBox.