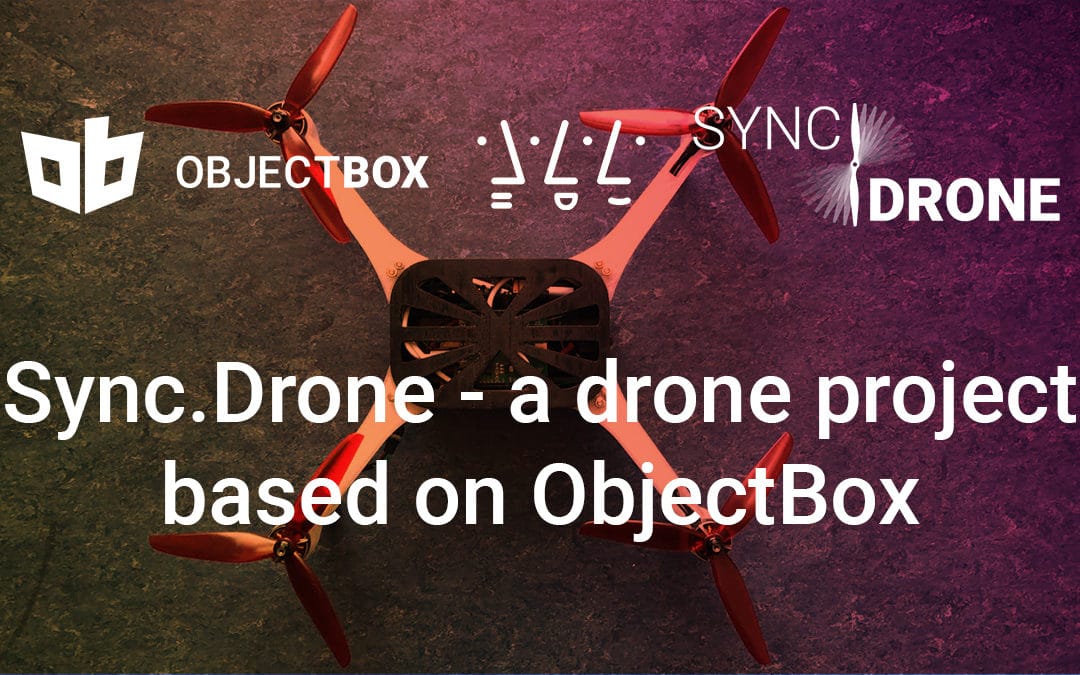
Sync.Drone: a drone in-flight synchronization project
This spring, a student group from Augsburg University of Applied Sciences build a drone application based on ObjectBox Database and Sync. This is a guest blog post by Michelle from the sync.drone project group, describing the project from start to finish and sharing the results.
The goal: Showcasing the ObjectBox database and Synchronization solution with drones
The goal of the project was to synchronize the colors and flight patterns between two drones coordinating the colours and flight formation autonomously in flight to showcase the ObjectBox Sync solution. Why? For a deeptech database startup it is often hard to demonstrate and communicate the uses of the technology. So, we, a team of students of the FH Augsburg went on a mission to help showcase. Due to ObjectBox’ speed, more data can be processed faster on each drone, saving resources, specifically battery. This allows drones to fly longer, but that really does not have that much sex appeal for a broader audience. However, adding some colorful lights and developing a special kind of light show… Going beyond the scope of a doable student showcase, the technology could be used to synchronize swarms of drones creating beautiful colored messages and patterns in the night sky. While our outlook was more on an artistic installation, such a showcase should also help transfer the application to other use cases, g.g. self-syncing drones could be used in emergency situations during a large-scale search for missing persons. Of course, there are more use cases in the future: Drones can also be used in large warehouses to facilitate the organization of different parts, and pass on the position of a particular part.
In this article, we will explore the process we used to build our self-synchronizing drones, sharing our software and hardware, so you can try it out yourself.
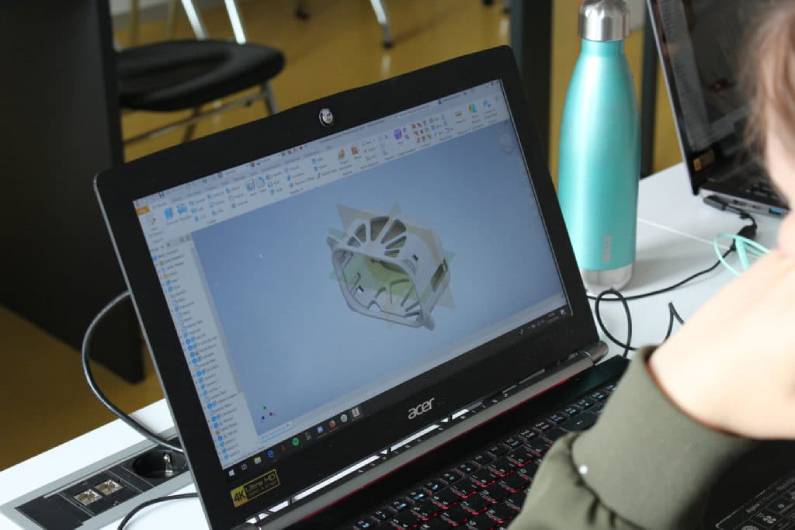
Hardware: Raspberry Pi, 3D printing, and more
In order to build our drones and turn our vision into reality, we had to consider a number of hardware options. It was important that our drone was compatible and programmable with ObjectBox. The drone had to be localizable and airworthy, so that a safe autonomous flight was possible. All parts had to be compatible so we could easily swap parts if something did not meet our requirements.
We built the drone frame from scratch, using 3D printers. The housing was created in the 3D program Autodesk Inventor and the parts were assembled to a drone frame. We used NeoPixel RGB LED sticks to make the drone glow in color. We chose the following components.
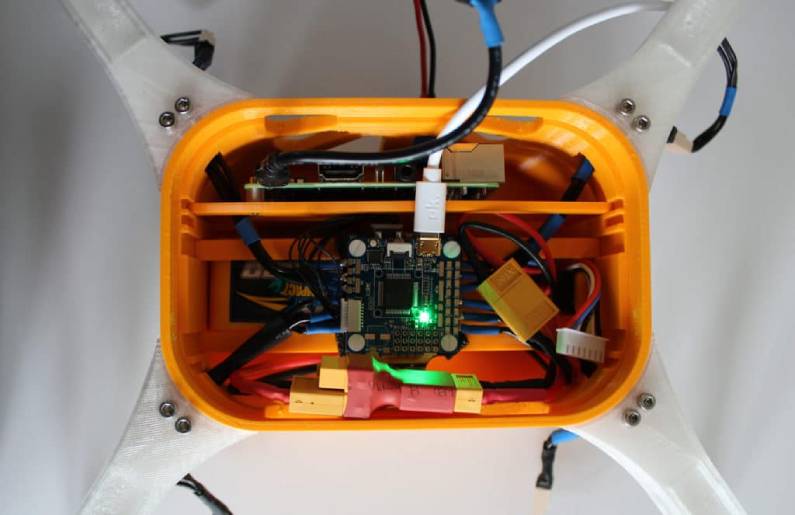
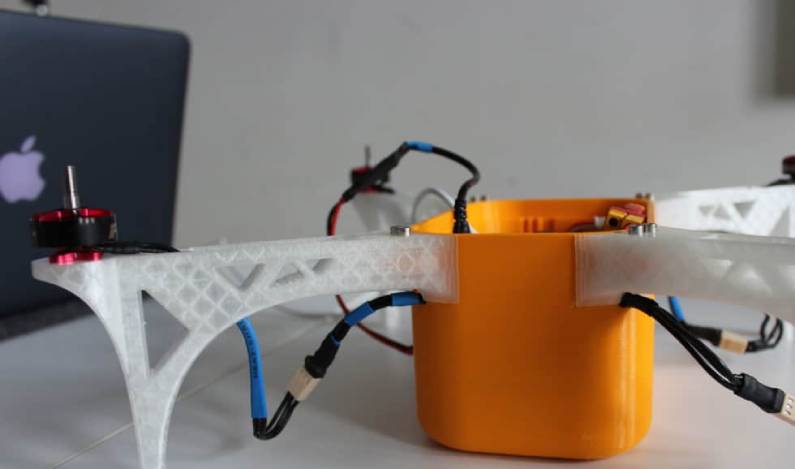
Microcomputer
A Raspberry Pi was the most suitable central computer on our drone. It offered both performance and size. We chose the Raspberry Pi 3 B+, which would later control the processes of our drone independently.
Tracking system
After looking at different tracking systems, we chose the “POZYX” UWB tracking system. This ensured an accurate and user-friendly handling.
Accumulator
We had to make sure that the drone’s battery would last long enough to power a Raspberry Pi, LEDs and a POZYX tag in addition to the flight hardware. First started with a 6 cell LiPo battery with 5000mAh. However, later in the project, we replaced the battery with a lighter and more compact 6-cell LiPo battery with 1800mAh.
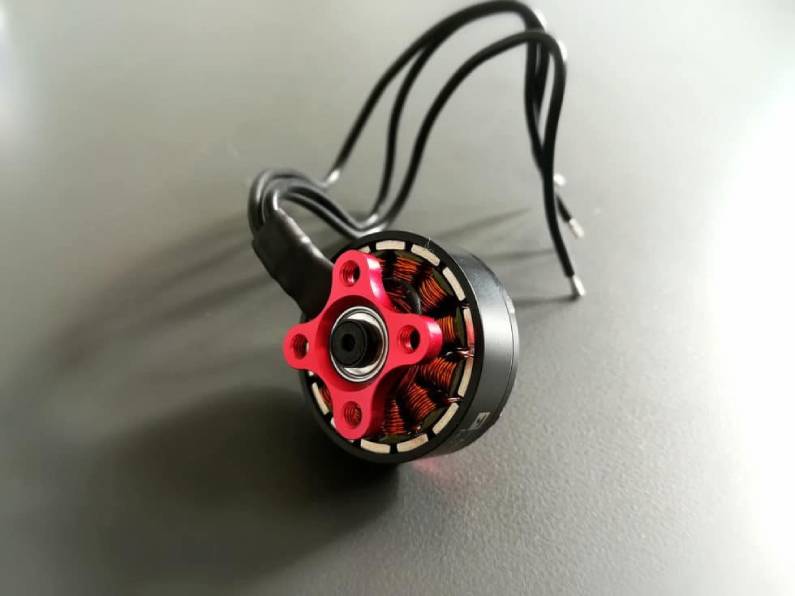
Engines
The engines (1750 kV) from the Drone-Racing sector had enough power to make the drone fly. Motors with even lower kV would have given the drone more power, but are much more expensive.
Flight controller
As flight controller we chose the “Omnibus F4 V6” chip, which ran with the open source software “Beta Flight” and was accessible via the so-called Multiwii Serial Protocol (MSP). This allowed us to use the advantages of a proven flight software, and also transfer the flight instructions via USB directly to the flight controller using the MSP.
Electronic Speed Controller (ESC)
For the ESC , which implements the instructions of the flight controller by direct voltage changes at the motors, we chose a 4-in-1 model. With only one connection cable to the flight controller, all four motors can be controlled at the same time. Usually one ESC is required per motor. It was also compatible with our hardware.
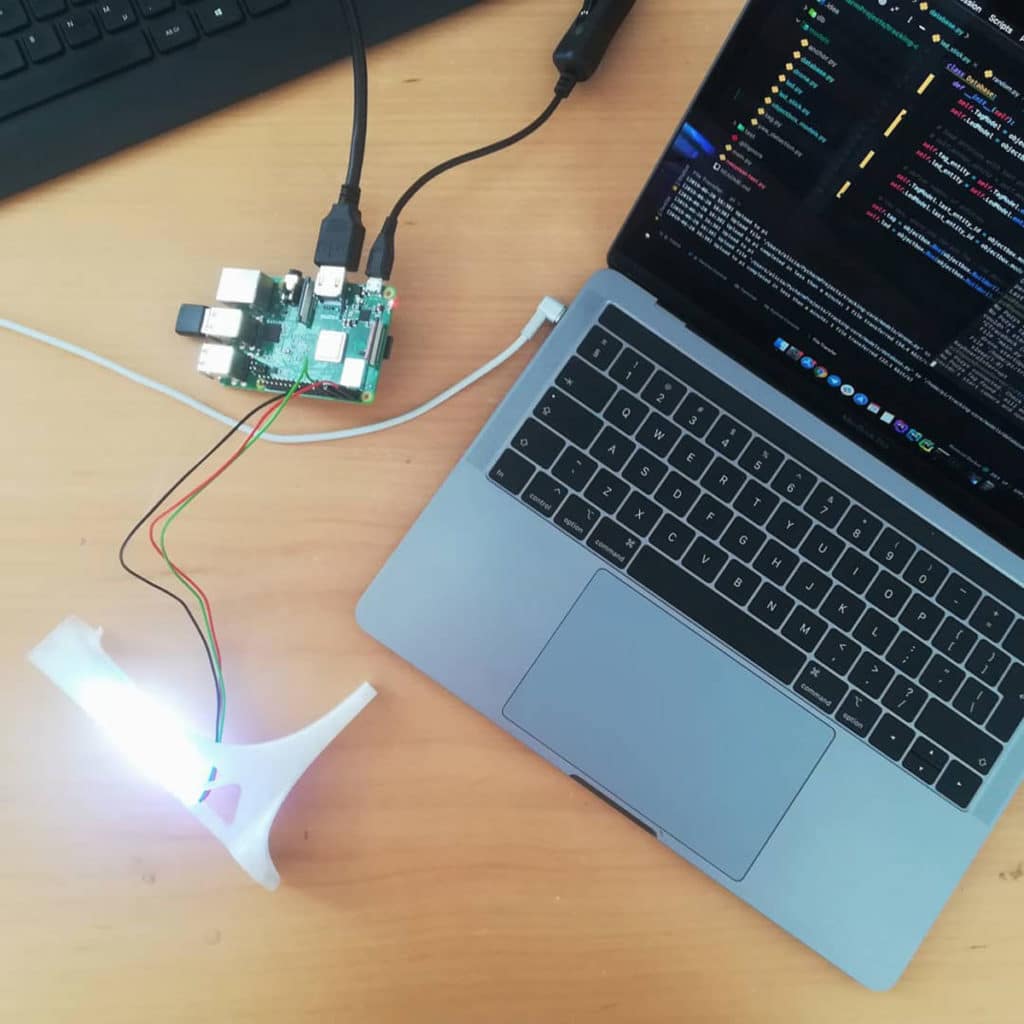
Software – Tracking, Flying and Syncing the Drones
Except for a start signal, the drone was supposed to operate without a remote control. Several drones would coordinate themselves at the same time according to the instructions. We decided to develop the code in three separate “cores”, which were merged at the end of the project. These were divided into “tracking”, “flying” and “syncing”. Using the university git lab as a repository, we were able to simplify development and share the code with the group. This allowed structured work on the code. With the help of ObjectBox and Prof. Dr. -Ing. Alexandra Teynor we were able to assemble the following code parts.
Tracking
For collision avoidance it was important to implement tracking, so that the drone knows it’s own position. We solved this by using the position calculated by the POZYX tag, which was then transmitted to the Raspberry Pi in the tracking core. We read the coordinates from the IMU sensors (“inertial measurement unit” = unit of measurement based on multiple sensors ) from the POZYX tag, but not the exact positioning.
The so called “heading”, or yaw of the drone, is read out by a magnetometer. However, this internal “compass” reacts to disruptive factors and can deliver inaccurate results. We solved the correction of the heading via an algorithm using OpenCV. This algorithm uses a small camera module on the drone and special markings on the ground to detect its orientation. This allows the direction vectors of the drones to be calculated more accurately.
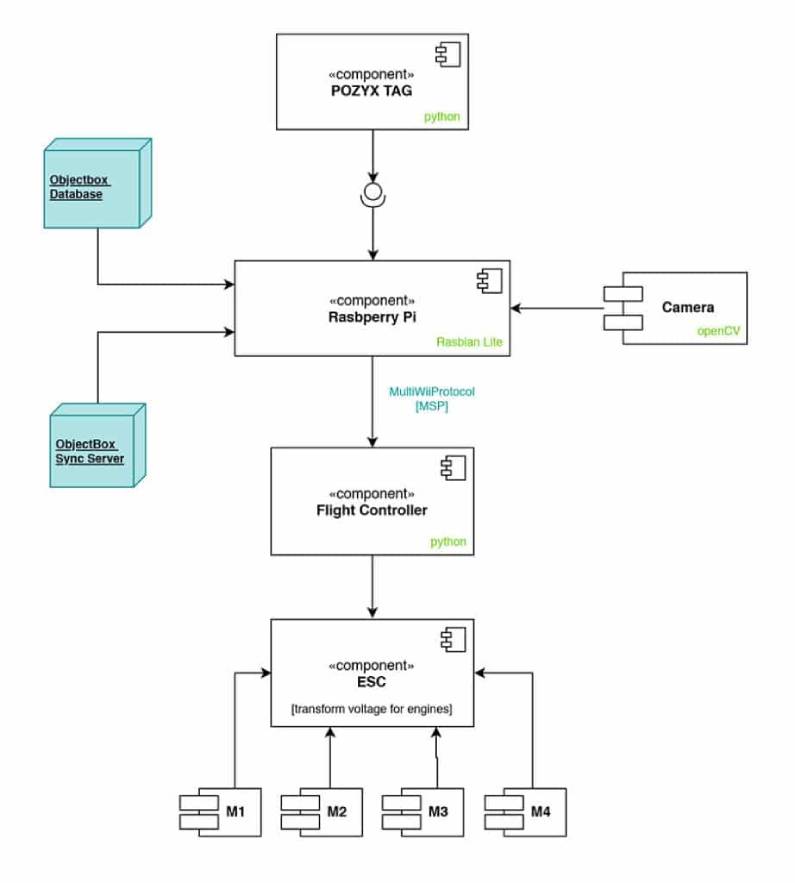
Flying
In the flying core, the flight instructions were developed based on the tracking core data, and then implemented by passing this data on to the flight controller. First of all the drones have to be lifted off the ground. For this purpose we used a laptop keyboard control, which forwarded flight instructions to the drone via a web socket.
Flight control
The Raspberry Pi establishes a serial connection to the flight controller via USB. As soon as this connection is established, flight instructions are transmitted in the form of inclination values for roll, pitch, yaw and throttle (thrust). These values may lie between 1000 and 2000. In a neutral position, roll, pitch and yaw are at an average value of 1500.
Using Python, we calculated the required roll, pitch, yaw and throttle values and assembled them using the Multiwii Serial Protocol. This was translated into pure byte code and sent to the flight controller via the USB cable. The flight controller now tries to reach the corresponding values. In order to turn to the right, the left motors are turned slightly up and the right motors slightly down. The ESC received the commands for the desired motor speed from the flight controller. It then applied the required voltage to each motor according to its instructions. The communication between the flight controller and ESC happened either by an analog (PWM) signal or a digital signal (D-Shot).
Keyboard control
The computer runs a Python script that registers keystrokes and converts them into instructions. For example, pressing the right arrow key creates the command “raise-roll” and pressing the left arrow key triggers the command “lower-roll”.
The drone also runs a Python script that opens a web socket to which the PC script connects. Each time a key is pressed on the laptop, a corresponding command string is generated (e.g. “raise-yaw”) and sent to the drone via the web socket. As soon as a string arrives, the relevant value (roll, pitch, yaw, throttle) is increased or decreased.
To prevent the drone from crashing if a connection is lost, the values are flattened algorithmically.
ObjectBox Database and Sync Drone Implementation
In the syncing core, the position data of all drones as well as the LED color, should be exchanged and commands passed on. The RGB color space of the LEDs was mapped to the x-, y- and z-position. In this way, the sync features of the drones could be displayed without them flying. For the implementation we used the ObjectBox database and the ObjectBox Sync Server.
Originally, we had planned to use the ObjectBox Go Binding because it is precompileable and very fast. However, the POZYX system we chose used Python. There was also already a Python implementation available for our flight controller, but none available for Go. Luckily, ObjectBox offered to develop and provide a small Python binding of their database according to our needs. This included all ObjectBox functions that were relevant to us. It was officially released in version 0.1.0 specifically for our project. As a result, the ObjectBox database could be easily integrated into our code.
Realization of syncing
In Python version 0.1.0, ObjectBox incorporated the basic features we needed. For our application the simple CRUD functions and the Sync feature, which synchronizes the data in near real time, were sufficient. The database is compact and the speed and ease of use is optimized for restricted IoT devices, for example the Raspberry Pi used in this project.
The sync server is started by running the init-server.py script on the master drone. At first, an empty database (model) was initialized. The master drone then communicates with the other drones via WLAN network connection and synchronizes the ObjectBox database between the respective devices.
1 2 3 4 5 6 7 8 |
import objectbox from models.objectbox_models import Led # initialize an empty DB before the first server start the server model = objectbox.Model() model.entity(Led, last_property_id=objectbox.model.IdUid(4, 3004)) model.last_entity_id = objectbox.model.IdUid(3, 3) model = objectbox.Builder().model(model).directory("led-server-db").build() |
Three entities (classes) are stored:
– the identification and position data of the anchors
– the identification and position data of the tags
– the color values of the LEDs.
The drone stored it’s position and LED color in the database. The master drone then reads out this information and overwrites it with the values calculated by the master drone (e.g. LED color or target position in the future).
1 2 3 4 5 6 7 8 9 10 11 12 13 14 15 |
from models.objectbox_models import Led as LedModel class Led: def __init__(self, database, tag_id): self.red = 0 self.green = 0 self.blue = 0 self.tag_id = tag_id self.db_id = database.led.put(LedModel()) self.led_object = database.led.get(self.db_id) def saveColorToDatabase(self, database): self.led_object.setColor(self.red, self.green, self.blue) database.led.put(self.led_object) self.led_object.printColor() |
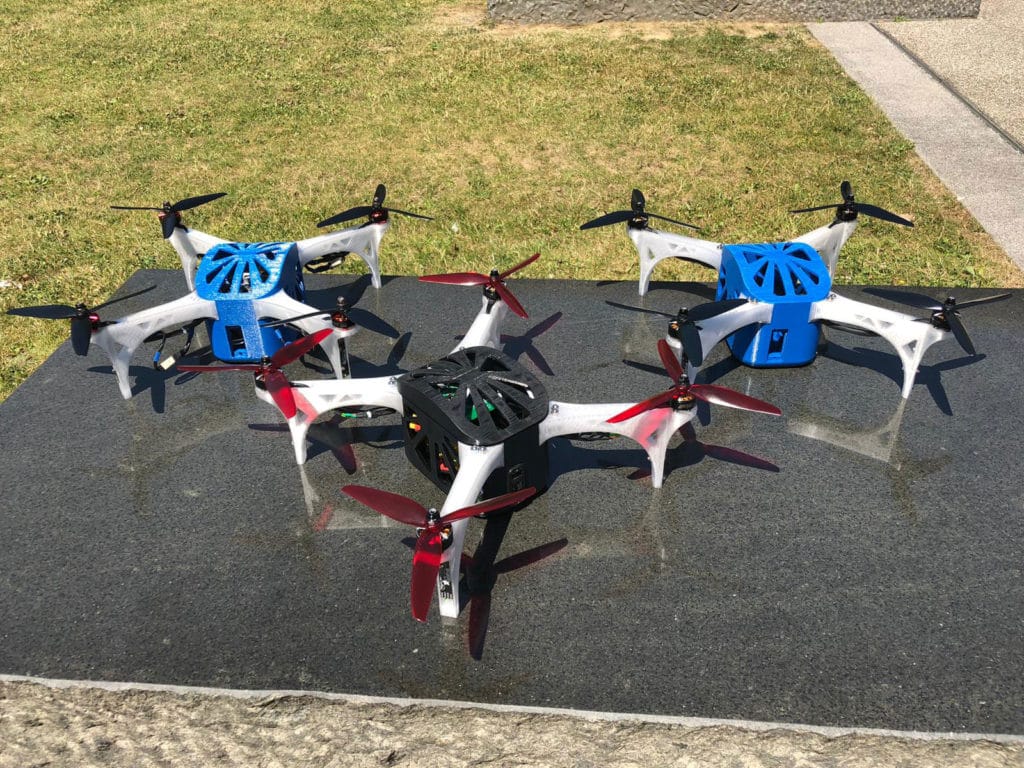
Thank you!
At the end of our project, we had three drones. Depending on the position of the master drone, all drones could synchronize their LED colors. Unfortunately we were not able to finish the flight due to a defect in the flight controller and a delayed delivery of parts. Finally we decided to publish the code for the drone control on GitHub. Additionally, you can get inspired on our website as well as on our social media platforms.
Furthermore, we would be happy, if the project would be continued by another group of students in the future. With our work we have created a basis for many more ideas. In summary, our project still has a lot of ambitious potential for the future.
Thanks to ObjectBox for this great opportunity – we mastered many problems along the way and learned a lot. Thanks for the constant support.We also thank our professors Prof. Dr. -Ing. Alexandra Teynor, Prof. KP Ludwig John and our coach Sandra Hobelsberger for their professional advice and patience. Finally, we would like to thank HSA_Innolab for their additional financial support and FabLab for their advice and resources.
In collaboration with interactive media students of the University of Applied Sciences in Augsburg.
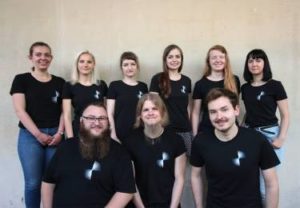
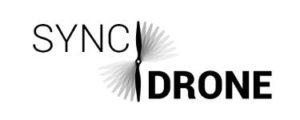
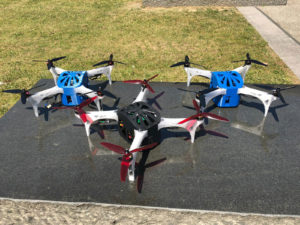
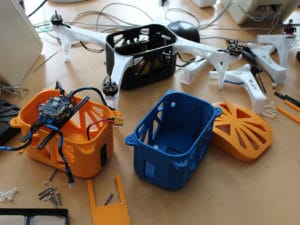
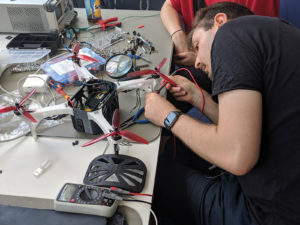